In the ever-evolving digital landscape, marketers face a constant challenge: how to deliver personalized experiences at scale while maintaining authenticity and relevance. Enter generative AI—a revolutionary technology that’s reshaping how brands connect with their audiences. As someone who has witnessed the evolution of digital marketing over the past two decades, I can confidently say that generative AI represents one of the most significant paradigm shifts since the advent of social media marketing.
Understanding Generative AI in Marketing
Generative AI refers to artificial intelligence systems capable of creating new content—text, images, videos, code, and more—based on patterns learned from training data. Unlike traditional rule-based systems, generative AI can produce original, contextually relevant content that feels remarkably human-like.
For marketers, this technology offers unprecedented capabilities to create personalized content, predict customer behavior, and optimize campaigns in ways that were previously impossible or prohibitively resource-intensive.
The Market Impact: Stats That Tell the Story
Before diving into specific applications, let’s look at some compelling statistics that highlight generative AI’s growing importance in marketing:
- According to McKinsey’s 2023 State of AI report, marketing is among the top three business functions seeing widespread AI adoption, with 66% of organizations reporting increased revenue after implementing AI-driven marketing strategies.
- Gartner predicts that by 2026, 60% of all marketing content will be generated or augmented by AI.
- A 2023 Salesforce study found that companies using generative AI for personalization see an average 25% increase in conversion rates and a 20% improvement in customer satisfaction scores.
- Research by Deloitte reveals that early adopters of generative AI in marketing report up to 40% reduction in content production costs while simultaneously increasing content output by 3-5x.
These numbers tell a compelling story: generative AI isn’t just a futuristic concept—it’s already delivering measurable value today.
Key Applications in Marketing and Personalization
1. Hyper-Personalized Content Creation
Traditional personalization often meant simply inserting a customer’s name into an email template. Generative AI takes this to an entirely new level by creating unique content tailored to individual preferences, behaviors, and contexts.
Real-World Example: Fashion retailer Stitch Fix uses generative AI to create personalized style recommendations that combine customer preferences, current trends, and stylist expertise. Their algorithms can generate written descriptions explaining why specific items might appeal to a customer based on their style profile, past purchases, and feedback. This approach has helped them achieve a remarkable 30% increase in customer retention compared to industry averages.
The content personalization process typically follows this workflow:
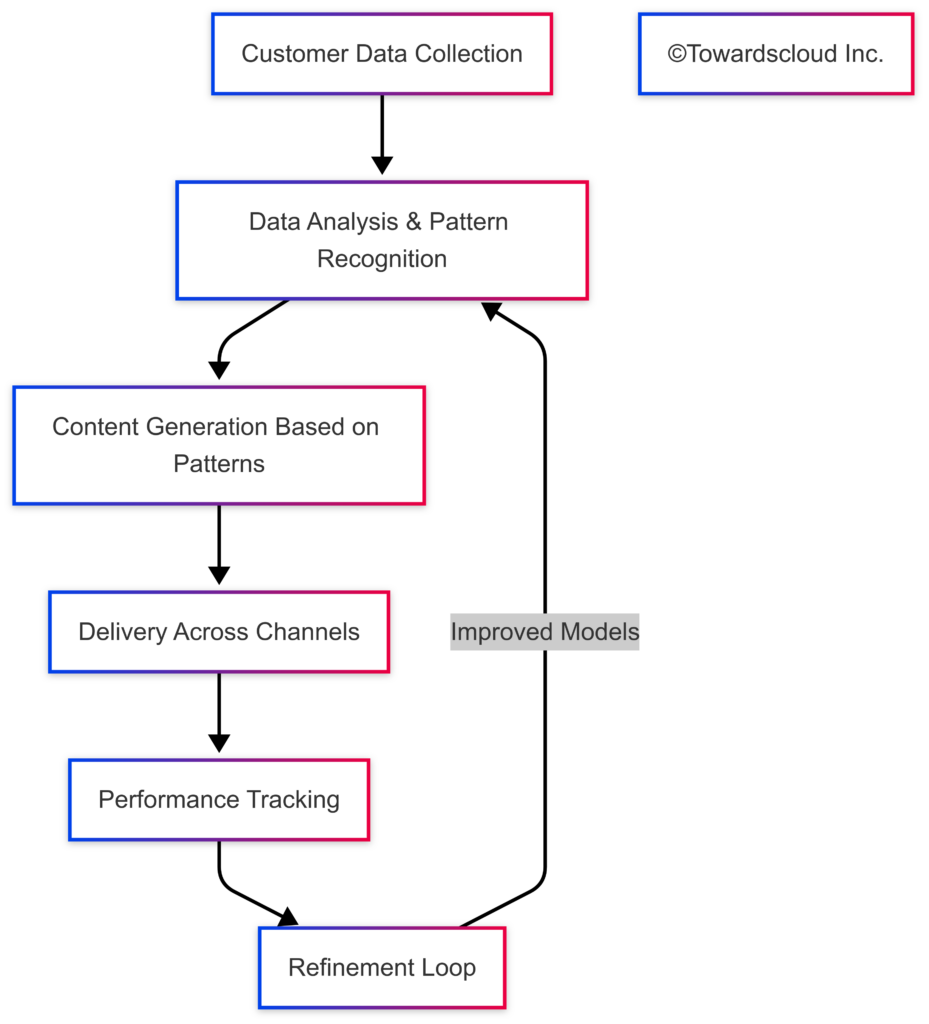
2. Dynamic Creative Optimization
Generative AI excels at producing and testing multiple creative variations simultaneously, enabling marketers to optimize visual assets, messaging, and calls-to-action in real-time based on performance data.
Real-World Example: Coca-Cola’s “Create Real Magic” campaign leveraged generative AI to produce thousands of unique creative assets. The system analyzed which visual elements resonated most with different audience segments and continuously optimized creative performance. This approach resulted in a 22% improvement in engagement rates compared to traditional campaigns.
The relationship between traditional and AI-enhanced creative processes looks something like this:
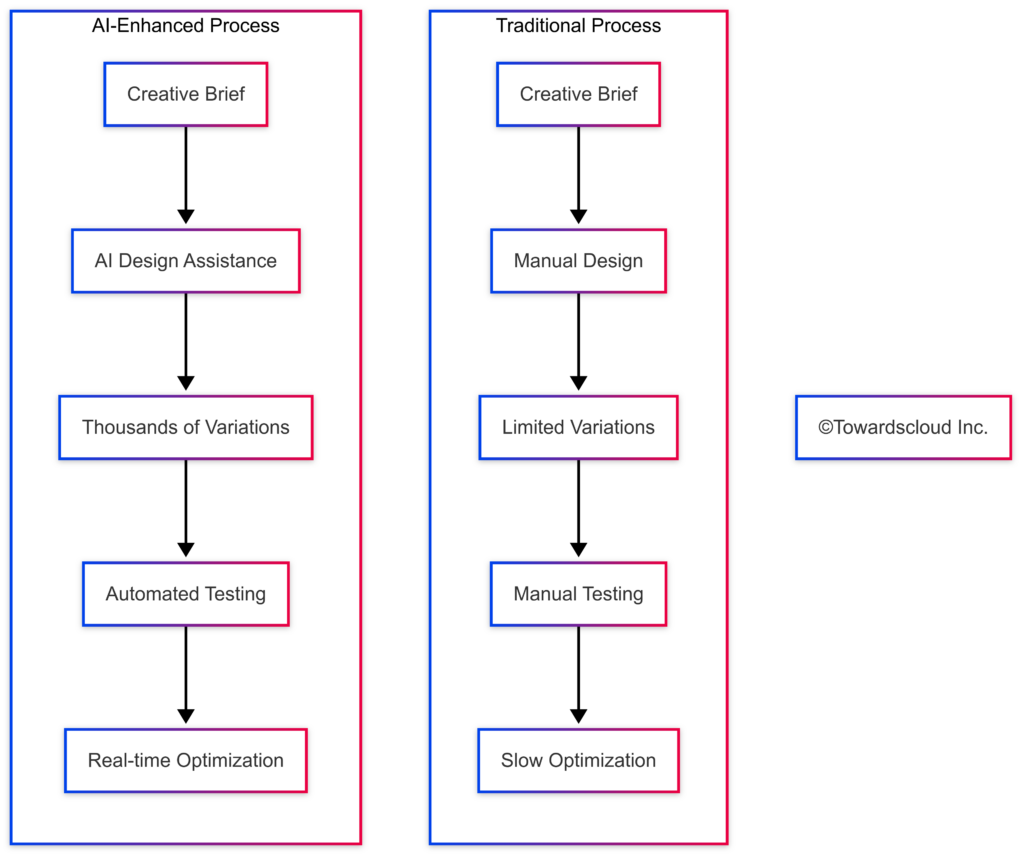
3. Conversational Marketing and Support
AI-powered conversational agents have evolved dramatically from simple rule-based chatbots to sophisticated assistants capable of nuanced interactions.
Real-World Example: Levi’s virtual stylist, powered by advanced language models, engages customers in natural conversations about fit preferences, style goals, and occasions. The system can generate personalized outfit recommendations with explanatory text about why certain pieces work well together, mimicking the experience of working with a human stylist. This implementation has increased average order value by 15% for users who engage with the AI stylist.
The evolution of conversational marketing tools illustrates the dramatic leap forward:
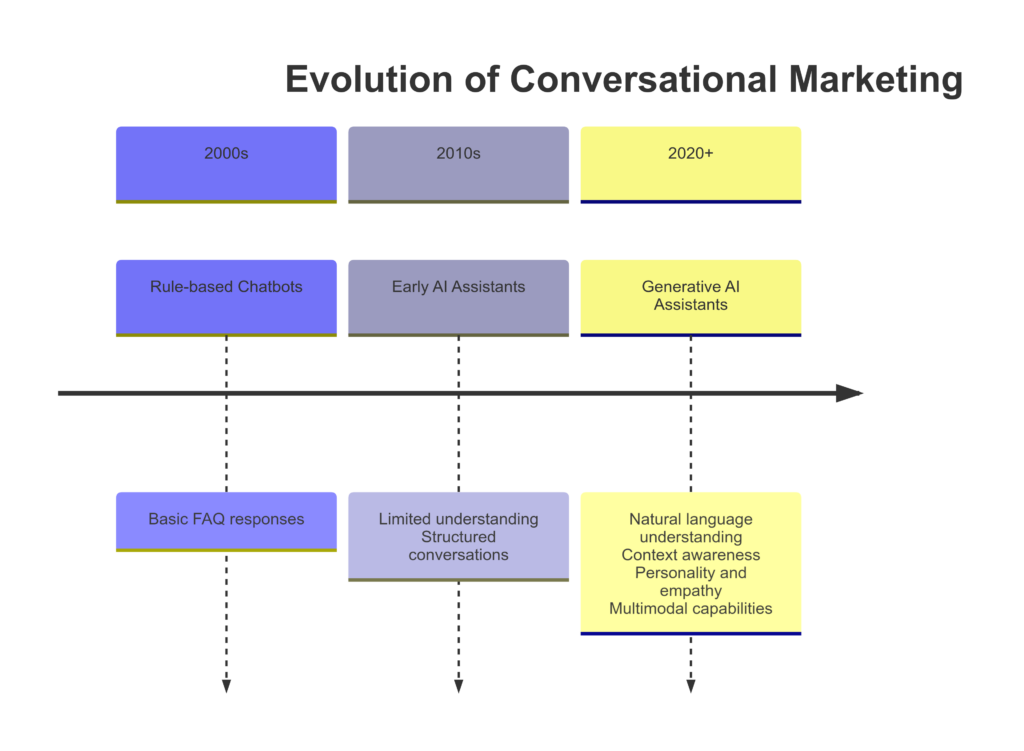
4. Predictive Customer Journey Mapping
By analyzing vast amounts of customer data, generative AI can predict future behaviors and preferences with remarkable accuracy, allowing marketers to anticipate needs rather than simply react to them.
Real-World Example: Streaming service Spotify uses generative AI not just for content recommendations but to predict entire listening journeys. Their system can generate personalized “audio days” that anticipate mood shifts throughout a user’s day, sequencing content in ways that feel intuitive and natural. This predictive approach has contributed to Spotify maintaining a churn rate significantly lower than industry averages in the competitive streaming space.
The AI-powered customer journey mapping process typically follows this pattern:
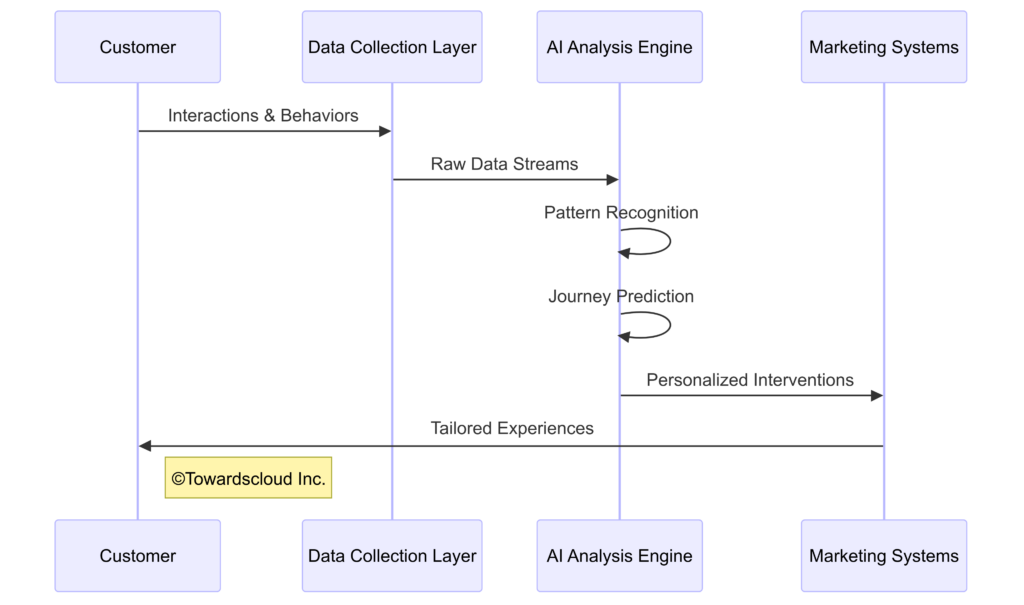
5. Dynamic Pricing and Promotion Optimization
Generative AI can continuously analyze market conditions, competitor pricing, inventory levels, and individual customer value to create personalized pricing and promotion strategies.
Real-World Example: Online travel agency Expedia uses generative AI to create personalized package offers that dynamically adjust pricing based on a complex matrix of factors including predicted customer lifetime value, current inventory, and competitive positioning. Their system can generate compelling package descriptions highlighting specific value propositions most likely to resonate with individual travelers. This approach has increased package booking rates by over 30% compared to static pricing models.
The complex decision tree that AI navigates for dynamic pricing looks something like this:
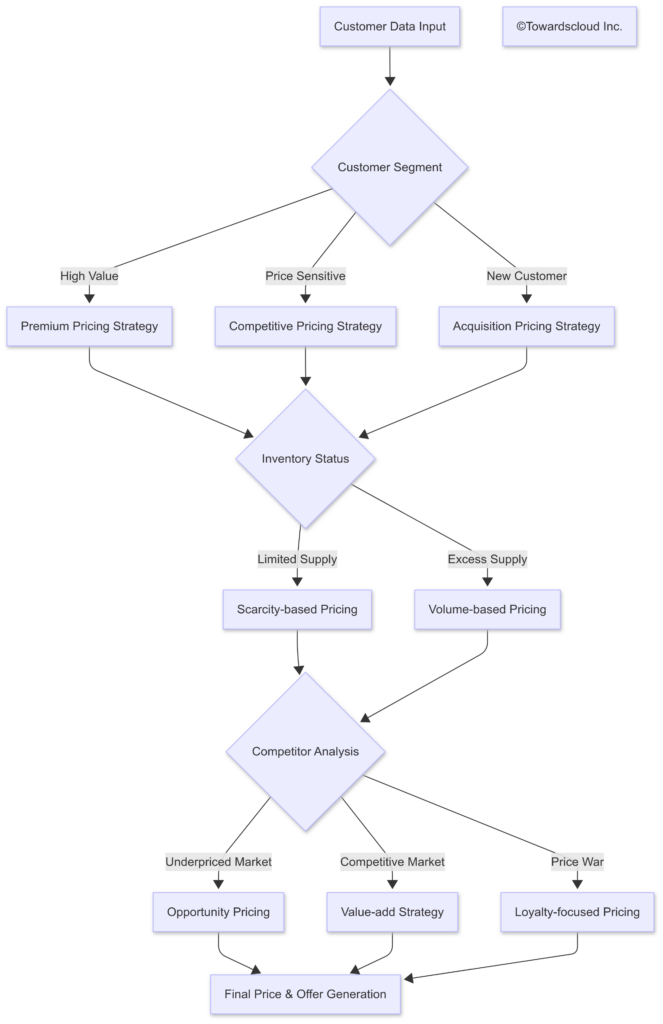
Implementation Approaches: Cloud Platform Comparison
Each major cloud provider offers distinct approaches to implementing generative AI for marketing and personalization. Here’s how they compare:
AWS Marketing AI Ecosystem
AWS provides a robust set of tools for marketing-focused AI applications:
- Amazon Personalize: Delivers real-time personalization and recommendations with minimal ML expertise required
- Amazon SageMaker Canvas: Enables no-code AI model building for marketing applications
- AWS Bedrock: Provides access to foundation models with marketing-specific fine-tuning options
AWS’s strength lies in its comprehensive data infrastructure and integration capabilities, making it particularly well-suited for organizations with complex data ecosystems and strict security requirements.
Google Cloud Marketing Solutions
Google Cloud brings unique marketing strengths:
- Vertex AI: Offers powerful generative AI capabilities with marketing-specific templates
- Recommendations AI: Delivers sophisticated product and content recommendations
- BigQuery ML: Enables predictive analytics directly within the data warehouse
Google’s marketing AI offerings benefit from the company’s deep expertise in consumer behavior and advertising, with particularly strong capabilities in search intent analysis and content optimization.
Microsoft Azure for Marketing
Azure’s marketing AI portfolio includes:
- Azure Personalizer: Learns and optimizes content selection to maximize user engagement
- Azure OpenAI Service: Provides access to powerful language models for content generation
- Azure Cognitive Services: Offers pre-built AI capabilities for sentiment analysis and content moderation
Microsoft’s strength is in enterprise integration and the familiar development environment for marketing teams already using Microsoft’s business tools.
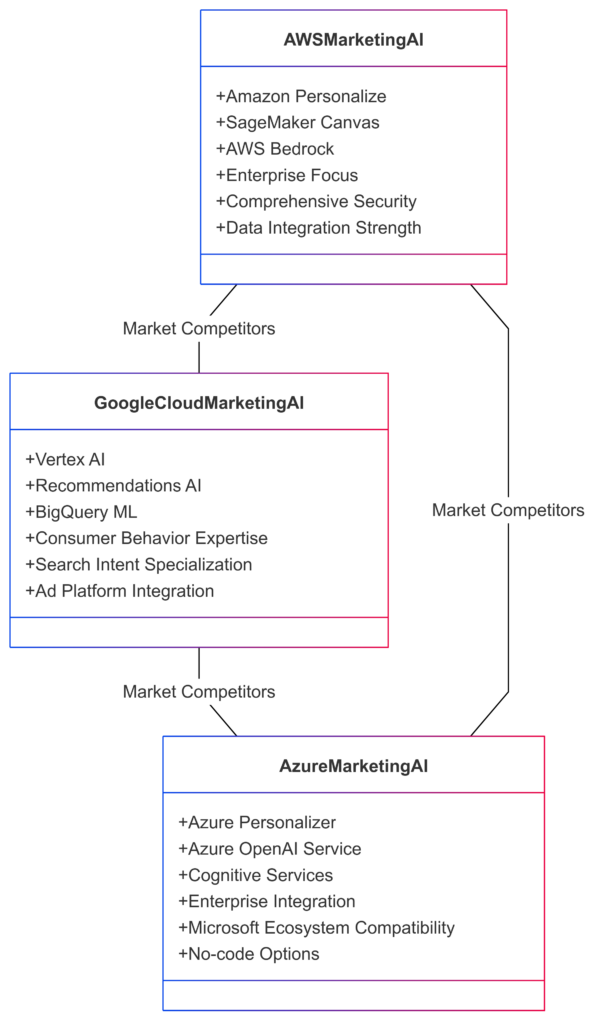
Ethical Considerations and Best Practices
The power of generative AI in marketing comes with significant responsibilities. Here are critical considerations for ethical implementation:
1. Transparency and Disclosure
Customers deserve to know when they’re interacting with AI-generated content or AI systems. Major brands like H&M and Sephora now explicitly label AI-generated content and clearly identify when customers are interacting with AI assistants rather than human agents.
2. Avoiding Harmful Biases
Marketing AI systems can perpetuate or amplify existing biases present in training data. Progressive organizations implement bias detection systems and diverse training data sets to mitigate these risks. Pinterest, for example, has implemented bias detection algorithms to ensure their AI-powered content recommendations don’t reinforce harmful stereotypes.
3. Data Privacy and Consent
With increasing regulatory scrutiny under frameworks like GDPR and CCPA, responsible marketers ensure their AI systems operate within clear consent frameworks. Apple’s approach to personalization demonstrates this balance, with transparent opt-in processes and on-device processing that minimizes data collection.
4. Human Oversight and Intervention
The most effective generative AI marketing implementations maintain human oversight, particularly for sensitive decisions or high-stakes content. Airbnb’s content moderation system combines AI flagging with human review teams, creating a powerful hybrid approach that leverages the strengths of both.
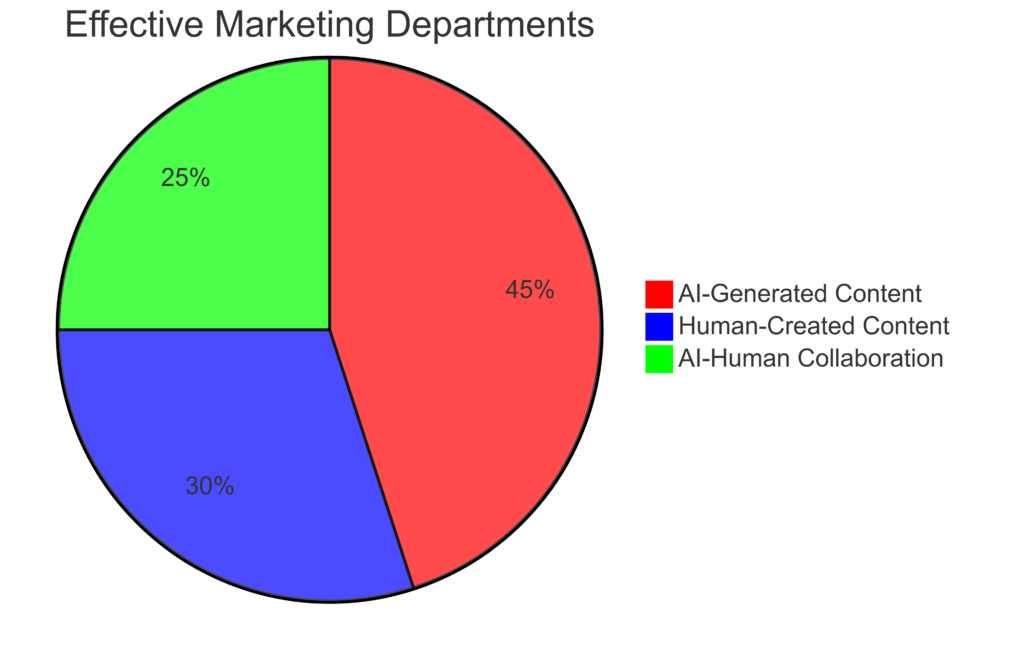
Implementation Roadmap for Marketers
For marketing teams looking to implement generative AI, I recommend this phased approach:
Phase 1: Foundation Building (1-3 Months)
- Audit Current Data Assets: Assess the quality, accessibility, and compliance status of your customer data
- Define Clear Use Cases: Identify specific problems where generative AI offers clear benefits
- Select Platform Partners: Evaluate which cloud platforms and specialized vendors align with your needs
Phase 2: Pilot Implementation (3-6 Months)
- Start with Narrow Applications: Focus on well-defined use cases with measurable outcomes
- Implement Testing Framework: Establish clear methods to compare AI-driven vs. traditional approaches
- Train Internal Teams: Build organizational capability to work effectively with AI systems
Phase 3: Scaling and Integration (6-12 Months)
- Expand Successful Use Cases: Apply learnings from pilots to broader implementation
- Integrate Across Channels: Create consistent AI-powered experiences across customer touchpoints
- Establish Governance Framework: Ensure ongoing ethical oversight and compliance
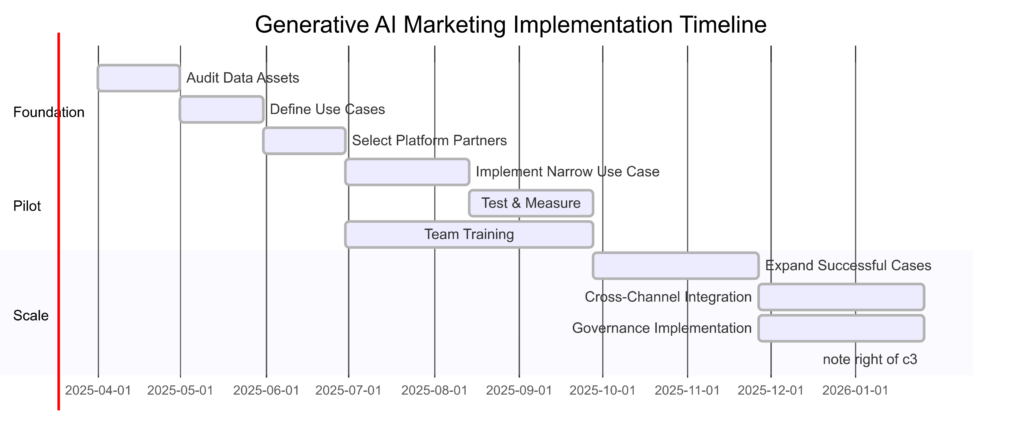
Future Trends to Watch
Looking ahead, several emerging trends will shape the future of generative AI in marketing:
1. Multimodal Personalization
The next frontier involves AI systems that seamlessly integrate text, image, audio, and video generation for truly immersive personalized experiences. Imagine product demonstrations automatically tailored to an individual’s specific use case and aesthetic preferences, delivered in their preferred communication style.
2. Emotion-Aware Marketing
Advanced sentiment analysis capabilities are evolving toward genuine emotion recognition, enabling marketing systems that can detect and respond appropriately to customer emotional states. This will transform everything from email marketing timing to service recovery interactions.
3. Zero-Party Data Optimization
As privacy concerns continue to reshape data collection practices, generative AI will play a crucial role in maximizing value from explicitly shared customer preferences. Systems will become increasingly adept at generating accurate predictions from minimal but high-quality data inputs.
4. Generative AI for Strategic Planning
Beyond tactical applications, generative AI is beginning to assist with higher-level marketing strategy development. Tools emerging in 2024 can analyze market conditions, competitive positioning, and internal capabilities to generate strategic recommendations and even draft comprehensive marketing plans.
5. Synthetic Data for Testing
As regulation increasingly restricts the use of customer data for experimentation, generative AI’s ability to create realistic synthetic datasets will become invaluable for testing marketing approaches without privacy concerns.
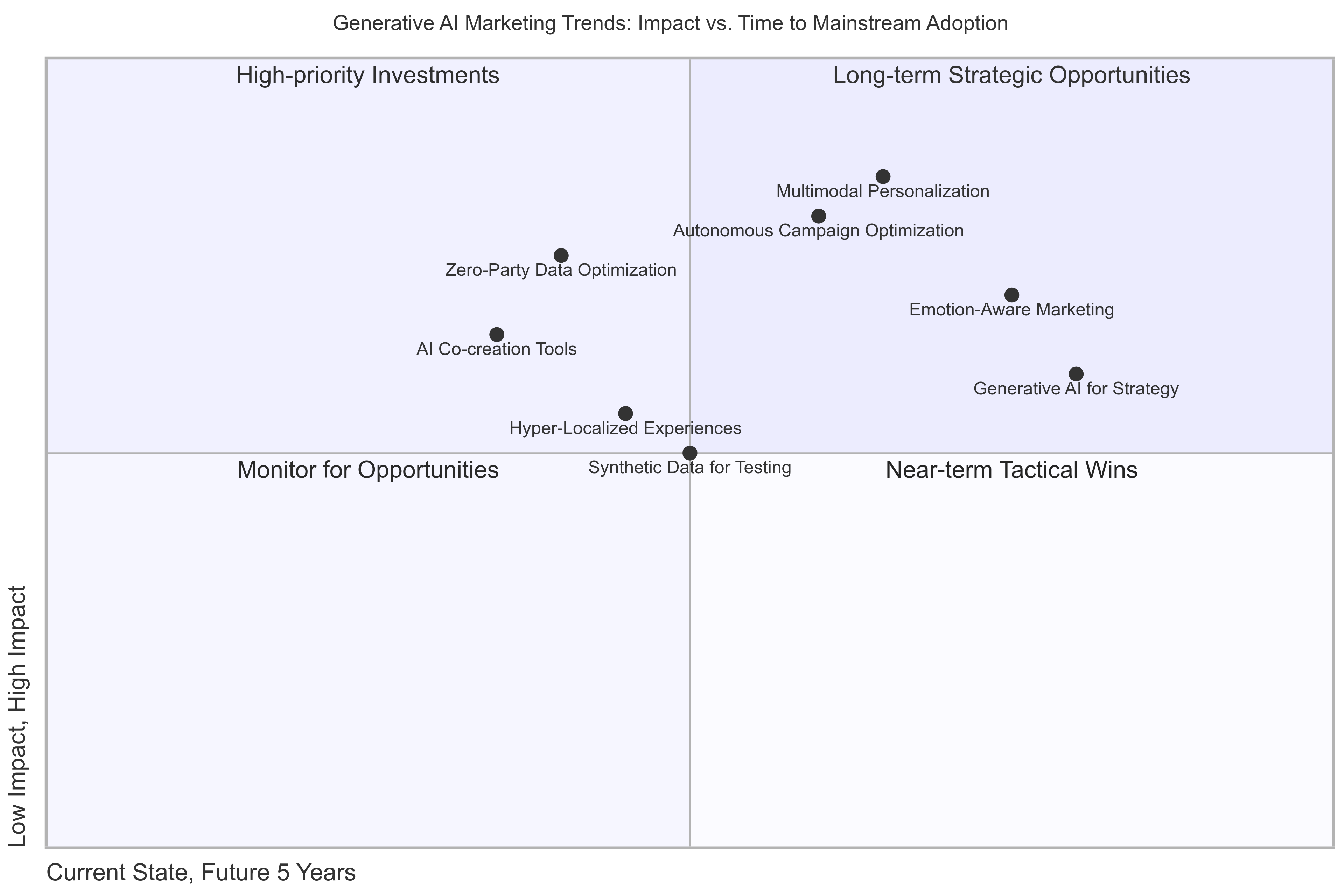
Conclusion
Generative AI represents a transformative force in marketing that extends far beyond mere automation or efficiency gains. It fundamentally changes what’s possible in personalization, creative production, and customer understanding. The technology enables marketers to deliver on the long-promised dream of truly individualized experiences at scale.
However, the most successful implementations will be those that thoughtfully integrate AI capabilities while maintaining human creativity, ethical oversight, and authentic brand voice. The brands that thrive in this new era won’t be those that simply deploy the most advanced AI, but those that find the right balance between technological capability and human connection.
As we move forward, marketers should approach generative AI with both enthusiasm for its possibilities and thoughtful consideration of its limitations and responsibilities. By focusing on clear use cases, building strong data foundations, and maintaining rigorous ethical standards, organizations can harness generative AI’s tremendous potential while avoiding its pitfalls.
The journey toward AI-enhanced marketing will continue to evolve, but one thing is certain: generative AI is not just another marketing technology trend—it represents a fundamental reshaping of how brands and customers connect in the digital age.
This article is part of our ongoing series exploring the intersection of cloud technologies and genAI innovations. For more insights on implementing cloud solutions, visit TowardsCloud.com.