Generative AI represents one of the most transformative technological developments in recent years. As cloud platforms rapidly integrate these capabilities into their service offerings, understanding both the technical and ethical dimensions becomes crucial for IT professionals implementing these powerful tools.
The Double-Edged Sword of Generative AI
Generative AI systems like ChatGPT, Claude, DALL-E, and Midjourney have democratized content creation in unprecedented ways. What once required specialized skills can now be accomplished through simple prompts. This accessibility, however, introduces significant ethical challenges that demand our attention.
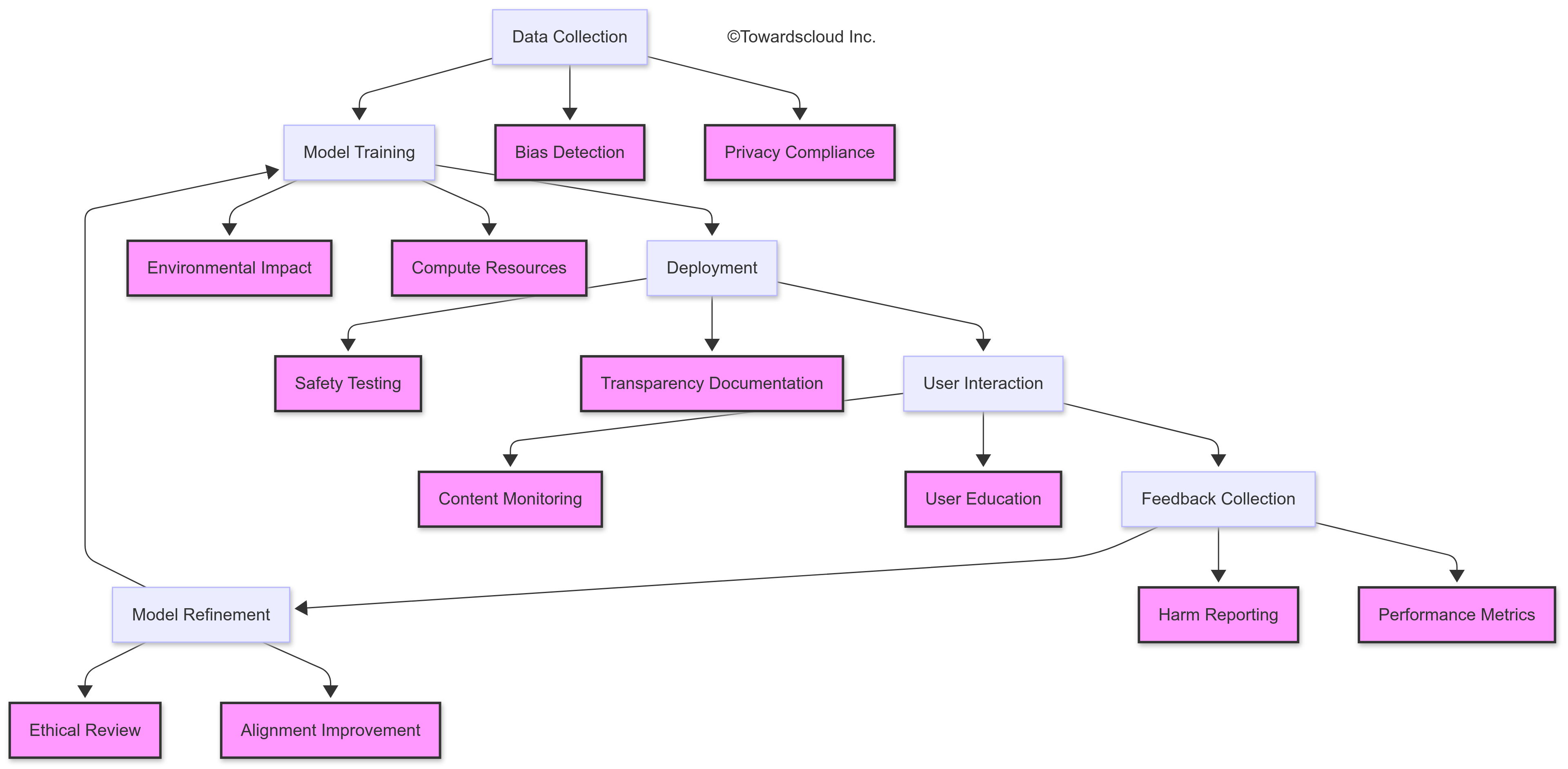
Bias and Representation
AI systems learn from existing data, inevitably absorbing the biases present in that data. Consider this real-world scenario: an HR department deployed a resume-screening AI that systematically downgraded candidates from certain universities simply because the training data reflected historical hiring patterns.
When implementing generative AI in AWS, you can use Amazon SageMaker’s fairness metrics to identify and mitigate bias. GCP offers similar capabilities through its Vertex AI platform, while Azure provides fairness assessments in its Responsible AI dashboard.
Content Authenticity and Attribution
The attribution challenges generative AI presents are significant. These systems don’t create truly original content—they synthesize patterns from existing works.
Best practices for using generative AI in content creation include:
- Clearly disclosing AI assistance
- Verifying factual claims independently
- Adding original insights and experiences
- Never presenting AI-generated content as solely human-created
Privacy Concerns
Training data often contains personal information. One engineering team discovered that their fine-tuned model was occasionally reproducing snippets of customer support conversations—a serious privacy breach.
Different cloud providers handle this differently:
- AWS SageMaker can be configured with VPC endpoints for enhanced data isolation
- GCP’s Vertex AI offers encrypted training pipelines
- Azure’s Machine Learning workspace provides robust data governance tools
Environmental Impact
The computational resources required for training large generative models are staggering. One training run of a large language model can emit more carbon than five cars produce in their lifetimes.
When selecting cloud providers for AI workloads, consider:
- GCP’s carbon-neutral infrastructure
- AWS’s commitment to 100% renewable energy by 2025
- Azure’s carbon negative pledge and sustainability calculator
Cloud Provider AI Ethics Comparison
AWS

Azure

GCP

Transparency and Explainability
As cloud professionals, we often deploy models we didn’t train ourselves. Understanding how these models make decisions is crucial for responsible implementation.
Azure’s Interpretability dashboard is particularly useful for understanding model behavior, while AWS provides SageMaker Clarify for similar insights. GCP’s Explainable AI offers feature attribution that helps identify which inputs most influenced an output.
Implementing Ethical Guardrails
Based on experience across AWS, GCP, and Azure, here are practical steps for ethical AI implementation:
- Document your ethical framework – Define clear principles and guidelines before deployment
- Implement robust testing – Test for bias, harmful outputs, and privacy violations
- Create feedback mechanisms – Enable users to report problematic outputs
- Establish human oversight – Never fully automate critical decisions
- Stay educated – This field evolves rapidly; continuous learning is essential
The Future of Responsible AI in Cloud Computing
All major cloud providers are developing tools for responsible AI deployment:
- AWS has integrated ethical considerations into its ML services
- Google’s Responsible AI Toolkit provides comprehensive resources
- Microsoft’s Responsible AI Standard offers a structured approach
Conclusion
As cloud professionals, we’re not just implementing technology—we’re shaping how it impacts society. The ethical considerations of generative AI aren’t separate from technical implementation; they’re an integral part of our professional responsibility.
What ethical considerations have you encountered when implementing generative AI in your organization? Share your experiences in the comments below.