In today’s rapidly evolving technological landscape, generative AI has emerged as a transformative force across industries. Healthcare, with its complex challenges and information-rich environment, stands to benefit tremendously from these advances. As we have observed the cloud computing and AI revolution unfold over decades, we are excited to explore how generative AI is reshaping healthcare and the unique considerations this intersection presents.
Understanding Generative AI in the Healthcare Context
Generative AI refers to artificial intelligence systems that can create new content – whether that’s text, images, code, or other data types – based on patterns learned from existing data. These systems use sophisticated deep learning models (often transformer-based architectures like GPT, LLaMA, or PaLM) to generate outputs that weren’t explicitly programmed.
In healthcare, generative AI is not just another technology trend; it represents a fundamental shift in how we can process, analyze, and derive value from medical information. Unlike traditional rule-based systems, generative AI can understand context, recognize patterns across disparate data sources, and even simulate creative problem-solving approaches.
Key Opportunities in Healthcare
1. Clinical Documentation and Administrative Efficiency
One of the most immediate applications of generative AI in healthcare is addressing the documentation burden that consumes up to 50% of physicians’ time. Recent studies have shown that implementing generative AI assistants can:
- Reduce documentation time by 33-40% on average
- Improve note quality and comprehensiveness
- Decrease physician burnout rates
- Free up an estimated 3-4 hours per day for direct patient care
Cloud providers have recognized this opportunity. AWS offers Amazon Comprehend Medical for natural language processing of clinical text, while Google Cloud’s Med-PaLM 2 can generate and analyze medical documentation with remarkable accuracy. Microsoft’s Azure OpenAI Service provides similar capabilities with fine-tuned healthcare models.
2. Enhanced Diagnostic Support
Generative AI is proving to be a powerful ally in diagnosis, particularly when integrated with imaging and lab data:
- Medical Imaging Analysis: AI systems can now not only detect anomalies in X-rays, CT scans, and MRIs but can generate detailed descriptions and differentials that assist radiologists.
- Multimodal Integration: The latest models can synthesize information across text, imaging, genomic, and laboratory data to suggest potential diagnoses that might otherwise be missed.
- Rare Disease Identification: By learning patterns across vast datasets, generative AI shows particular promise in identifying rare conditions that human physicians might not encounter in their normal practice.
A 2023 study in Nature Medicine demonstrated that a multimodal generative AI system achieved 91% accuracy in diagnosing complex respiratory conditions – outperforming general practitioners by 17 percentage points while providing detailed explanations for its reasoning.
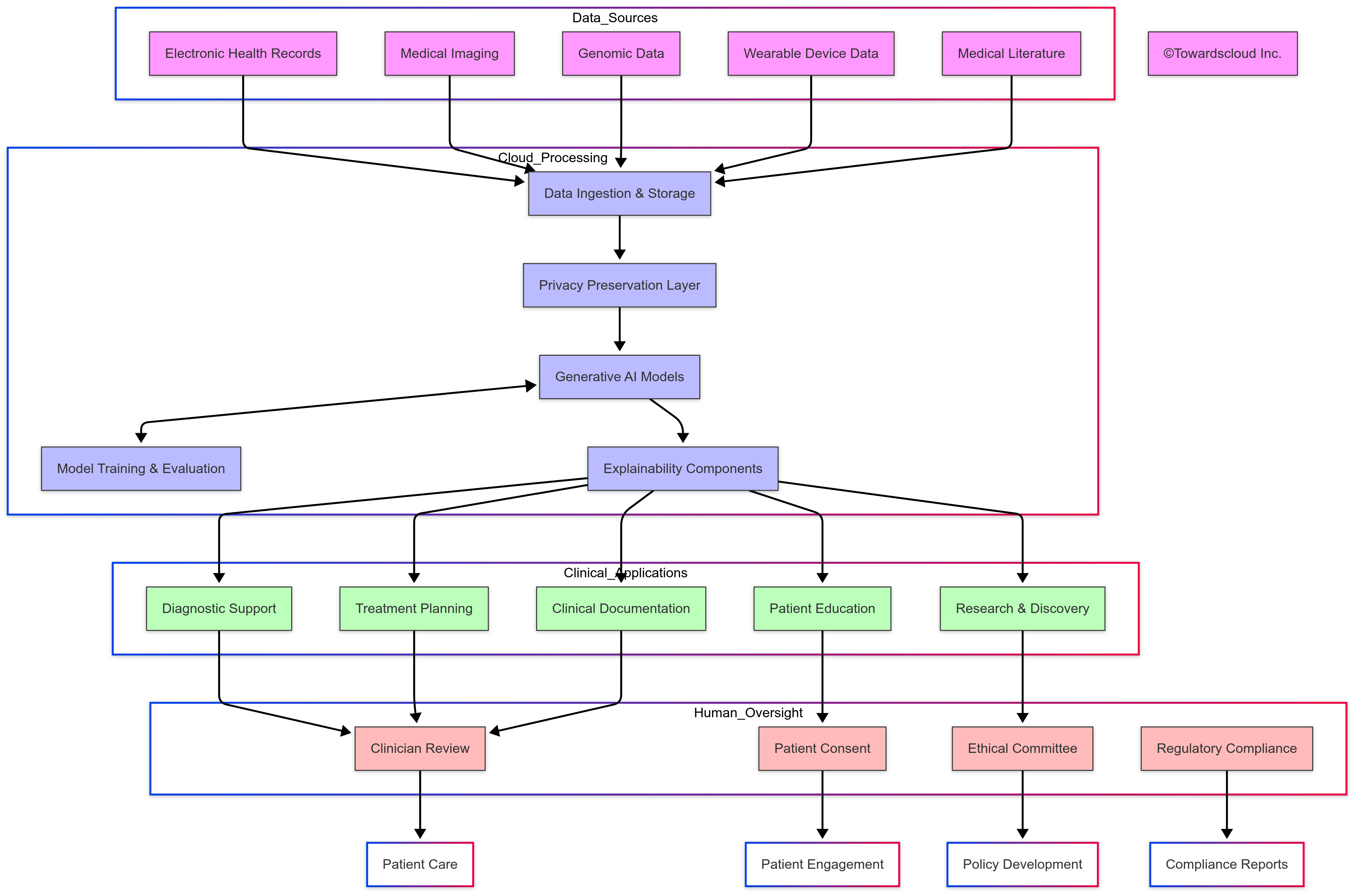
3. Personalized Treatment Planning
The generative capabilities of modern AI extend to treatment planning:
- Customized Treatment Protocols: By analyzing millions of patient records, generative AI can suggest personalized treatment plans based on similar cases and outcomes.
- Drug Discovery and Repurposing: AI systems like DeepMind’s AlphaFold and Isomorphic Labs’ tools are revolutionizing how we discover and repurpose medications by generating potential molecular structures.
- Clinical Trial Matching: Generative AI can identify suitable clinical trials for specific patients and even help design more effective trial protocols.
4. Patient Engagement and Education
Healthcare is increasingly consumer-driven, and generative AI is transforming the patient experience:
- Conversational AI: Azure Health Bot and similar tools enable natural language interactions that can answer patient questions, provide personalized health information, and guide them through care processes.
- Tailored Health Education: Generative AI can create customized educational materials that match a patient’s specific condition, literacy level, and learning preferences.
- Remote Monitoring Support: AI assistants can interpret data from wearables and home monitoring devices, providing meaningful insights and recommendations to patients.
Implementation Considerations Across Cloud Platforms
Each major cloud provider offers distinct approaches to healthcare generative AI implementation:
AWS Healthcare AI Ecosystem
AWS provides a comprehensive stack for healthcare generative AI:
- AWS HealthLake: HIPAA-eligible service that stores and analyzes health data in FHIR format
- Amazon Bedrock: Provides access to foundation models with healthcare-specific fine-tuning options
- SageMaker Canvas: Enables no-code AI model building for healthcare workflows
AWS’s approach emphasizes security compliance and integration with existing healthcare data ecosystems, making it particularly suitable for established healthcare organizations with strict regulatory requirements.

Google Cloud Healthcare Solutions
Google Cloud brings unique strengths to healthcare AI:
- Med-PaLM 2: Specialized medical language model designed to handle healthcare queries with high accuracy
- Healthcare Natural Language API: Extracts medical knowledge from unstructured text
- Healthcare Data Engine: Harmonizes disparate healthcare data sources for AI applications
Google’s healthcare AI offerings benefit from the company’s deep research expertise and tend to excel at natural language understanding of complex medical concepts.
Microsoft Azure for Healthcare
Azure’s healthcare AI portfolio includes:
- Azure Health Data Services: Manages diverse healthcare data types in a unified platform
- Azure OpenAI Service: Provides access to powerful language models with healthcare fine-tuning
- Azure AI Studio: Enables creation of healthcare-specific AI solutions with built-in governance
Microsoft’s strength lies in enterprise integration and the familiar development environment for healthcare IT teams already using Microsoft tools.
Critical Challenges and Ethical Considerations
Despite the promising opportunities, several significant challenges must be addressed:
1. Data Privacy and Security
Healthcare data is exceptionally sensitive, making privacy concerns paramount:
- HIPAA Compliance: All generative AI deployments must meet strict healthcare data protection standards
- De-identification Challenges: Even “anonymized” data can sometimes be re-identified through sophisticated means
- Model Inversion Attacks: Theoretical risk of extracting training data from models
- Cross-border Data Governance: Different jurisdictions have varying healthcare data protection requirements
Each cloud provider approaches these challenges differently, with AWS offering HIPAA-eligible services by default, Google emphasizing technical safeguards, and Microsoft providing comprehensive compliance documentation.
2. Bias and Fairness
Healthcare AI systems can perpetuate or amplify existing biases:
- Training Data Disparities: Historical medical data often underrepresents certain populations
- Algorithmic Bias: Models may develop unintended biases that affect care recommendations
- Access Inequality: Benefits of AI may not reach underserved communities equally
Recent research from Stanford’s HAI (Human-Centered AI) institute found significant performance disparities in generative AI systems across different demographic groups for certain medical conditions, highlighting the ongoing challenge of building fair healthcare AI.
3. Clinical Validation and Regulatory Approval
The path to widespread clinical implementation involves significant regulatory hurdles:
- FDA Oversight: Generative AI applications may require FDA clearance as medical devices
- Clinical Validation: Rigorous testing is needed to ensure real-world effectiveness
- Liability Questions: Unclear responsibility frameworks when AI contributes to care decisions
- Evolving Regulatory Landscape: Agencies are still developing frameworks for generative AI oversight
4. Explainability and Trust
Healthcare decisions require transparency that many AI systems struggle to provide:
- Black Box Problem: Complex generative models often can’t explain their reasoning clearly
- Clinician Acceptance: Healthcare professionals need to trust AI recommendations
- Patient Understanding: Patients deserve to know how AI influences their care
- Audit Trails: Maintaining records of AI-influenced decisions for review and improvement
Implementation Best Practices
For healthcare organizations considering generative AI adoption, I recommend:
- Start with Well-Defined Use Cases: Focus on specific problems where generative AI offers clear benefits and existing processes are well understood.
- Implement Robust Governance: Establish clear policies for data usage, model training, and deployment oversight before implementation begins.
- Prioritize Human-AI Collaboration: Design systems that enhance rather than replace human expertise, with appropriate oversight at critical decision points.
- Measure Impact Comprehensively: Evaluate not just technical performance but clinical outcomes, user experience, and unintended consequences.
- Build Cross-Functional Teams: Successful implementation requires clinical, technical, ethical, and operational expertise working together.
Future Directions
Looking ahead, several trends will shape healthcare generative AI:
- Multimodal Integration: Future systems will seamlessly combine text, images, genomic data, and more.
- Federated Learning: Training models across institutions without sharing sensitive data will become standard practice.
- Edge Deployment: More AI capabilities will run locally on devices to improve privacy and reduce latency.
- Regulatory Maturation: Clearer frameworks for healthcare AI approval and monitoring will emerge.
- Specialized Healthcare Models: Models specifically designed for healthcare, rather than adapted from general-purpose AI, will proliferate.
Conclusion
Generative AI represents a paradigm shift for healthcare with the potential to enhance care quality, improve efficiency, and create more personalized patient experiences. However, responsible implementation requires careful navigation of complex ethical, technical, and regulatory challenges.
As cloud platforms continue to develop specialized healthcare AI offerings, organizations must thoughtfully evaluate which approaches best align with their specific needs, values, and infrastructure. The most successful implementations will be those that thoughtfully integrate AI capabilities into existing workflows while maintaining human judgment at the center of critical care decisions.
The journey toward AI-enhanced healthcare will be neither simple nor linear, but the potential benefits for patients, providers, and health systems make it a worthwhile endeavor. By approaching these technologies with both optimism and careful consideration of their limitations, we can harness generative AI’s transformative potential while safeguarding the human connections at healthcare’s core.
This article is part of our ongoing series exploring the intersection of cloud technologies and genAI innovation. For more insights on implementing cloud solutions in regulated environments, visit TowardsCloud.com.