Imagine a world where machines can create art, compose music, and even write stories. This isn’t science fiction—it’s the reality of Generative AI. But how did we get here? Join us on a journey through the fascinating history of Generative AI, from its conceptual origins to the groundbreaking technologies shaping our world today.
Introduction
Generative AI, a subset of artificial intelligence, focuses on creating new content, from text and images to music and even entire virtual worlds. This blog will explore the key milestones, technologies, and pioneers that have brought Generative AI from a mere concept to a transformative reality.
The Early Days: Conceptual Foundations
1. The Birth of AI (1950s-1960s)
The journey of Generative AI begins with the birth of artificial intelligence itself. In the 1950s, pioneers like Alan Turing and John McCarthy laid the groundwork for AI. Turing’s famous question, “Can machines think?” and his development of the Turing Test set the stage for future explorations in machine creativity.
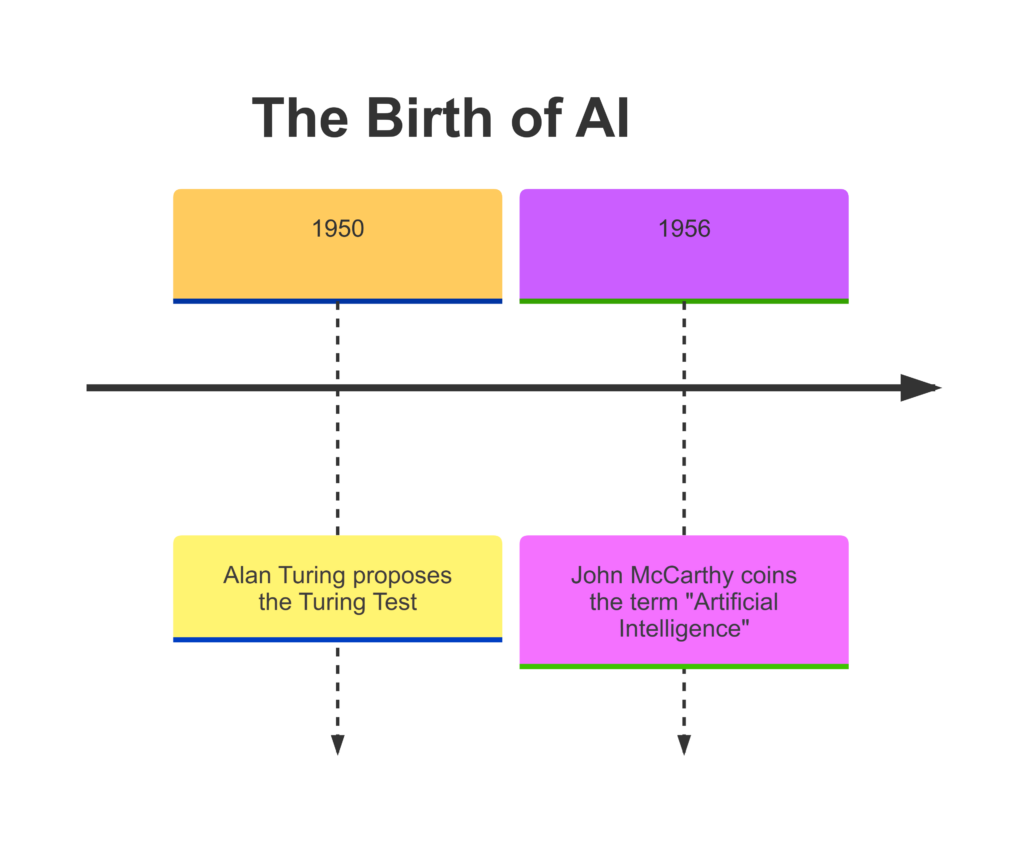
2. Early Experiments in Machine Creativity (1970s-1980s)
In the 1970s and 1980s, researchers began experimenting with algorithms that could generate simple forms of content. One of the earliest examples was the ELIZA program, which could simulate a conversation by generating text responses.
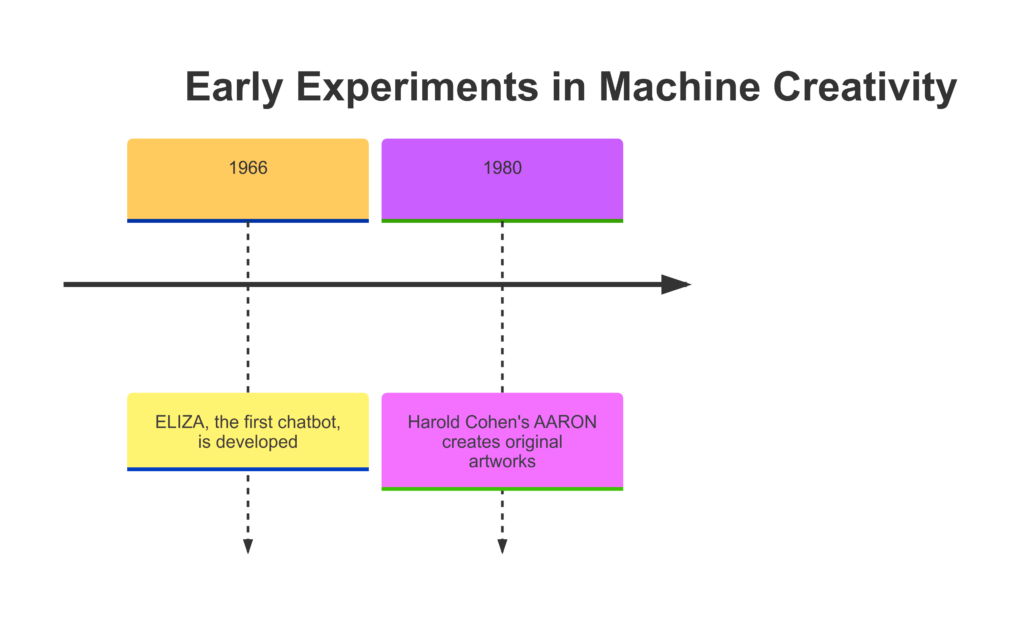
The Rise of Machine Learning (1990s-2000s)
3. The Emergence of Neural Networks (1990s)
The 1990s saw the rise of neural networks, which mimic the human brain’s structure and function. These networks became the foundation for modern Generative AI. Researchers began to explore how these networks could be trained to generate new data.
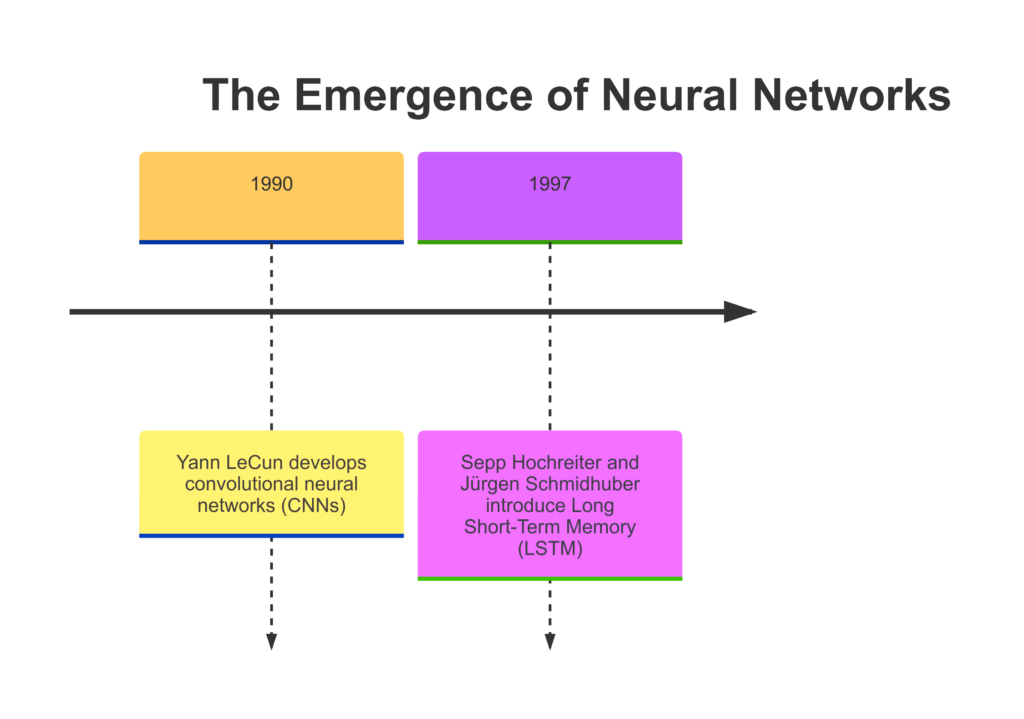
4. The Advent of Generative Models (2000s)
In the 2000s, the development of generative models like Restricted Boltzmann Machines (RBMs) and Generative Adversarial Networks (GANs) marked a significant leap forward. GANs, introduced by Ian Goodfellow in 2014, became a cornerstone of Generative AI.
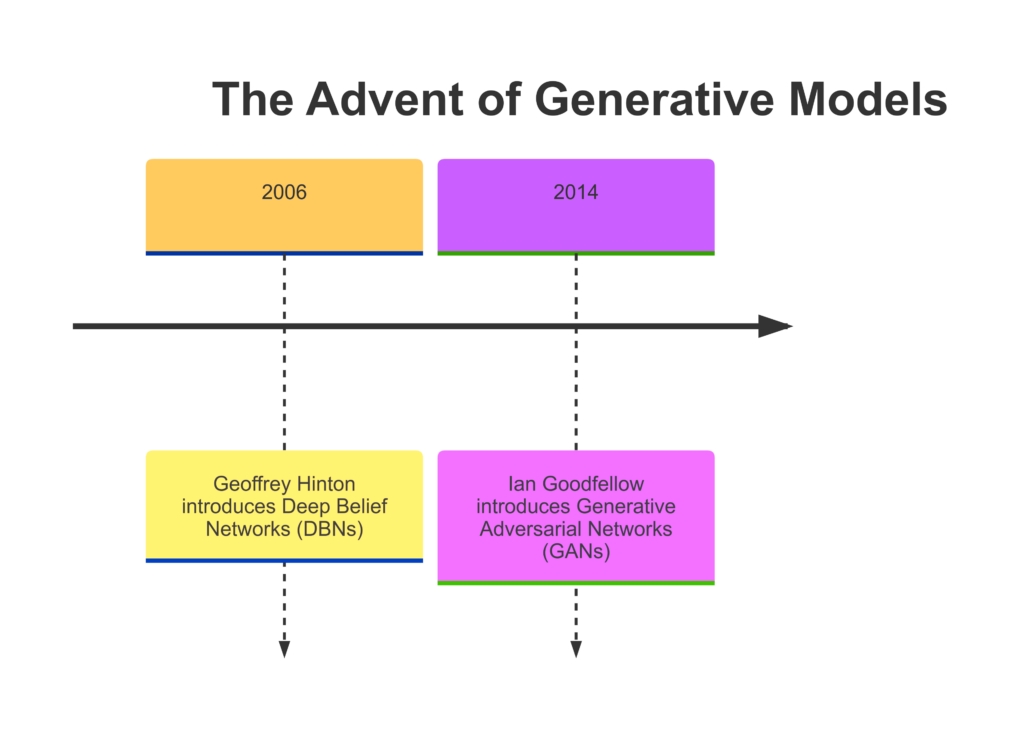
The Modern Era: Breakthroughs and Applications (2010s-Present)
5. The Deep Learning Revolution (2010s)
The 2010s were marked by the deep learning revolution, fueled by advancements in computational power and the availability of large datasets. This era saw the rise of models like OpenAI’s GPT series, which could generate human-like text.
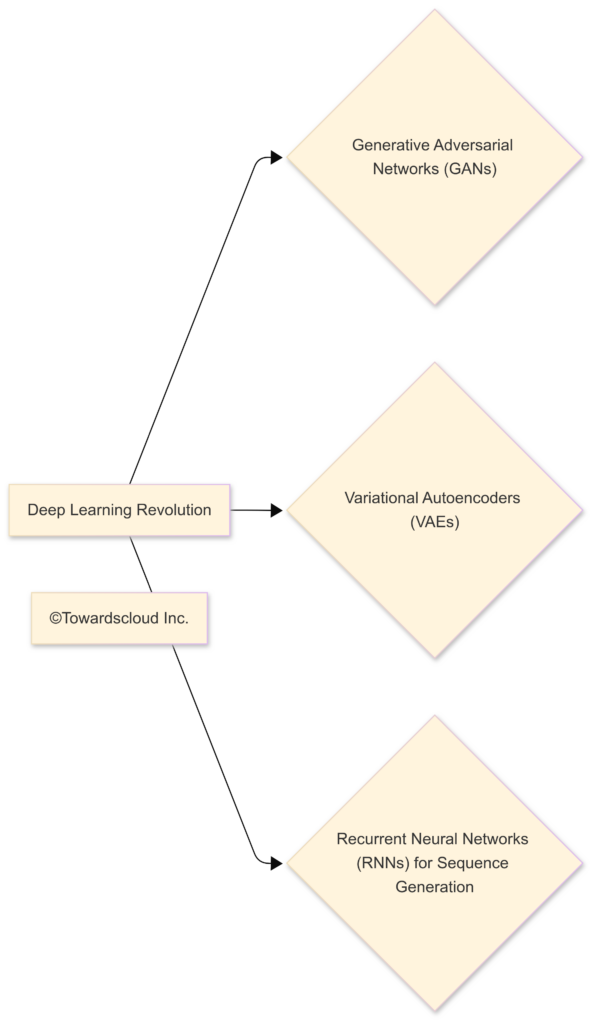
6. Generative AI in the Real World (2020s)
Today, Generative AI is transforming industries. From creating realistic images and videos to generating code and even designing drugs, the applications are endless. Models like DALL-E, MidJourney, and ChatGPT are pushing the boundaries of what machines can create.
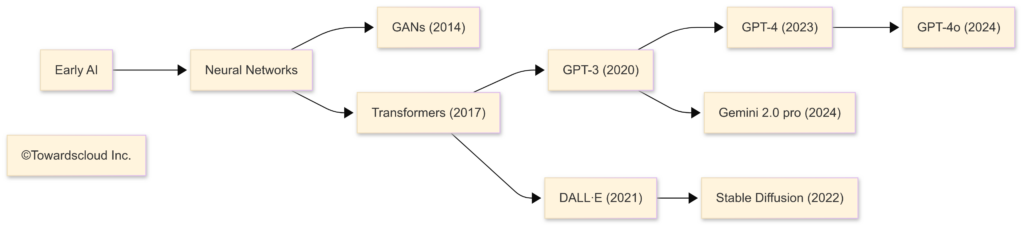
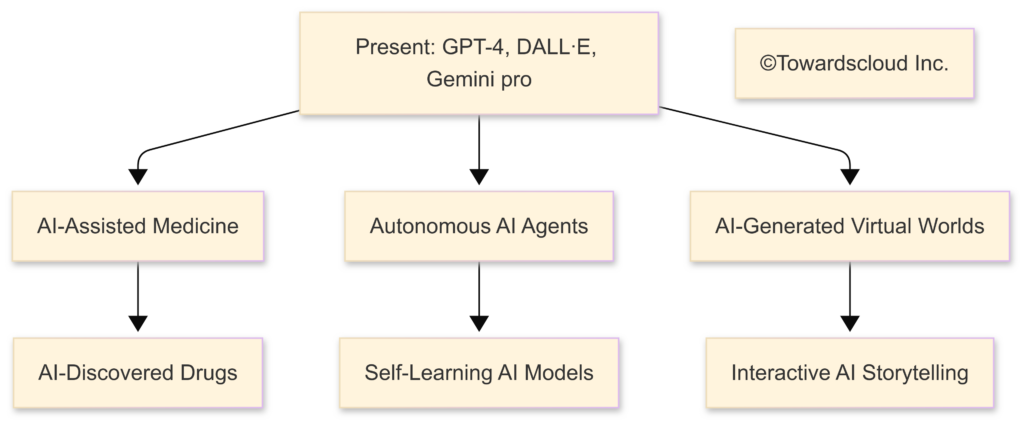
Engage with Us!
What excites you most about the future of Generative AI? Share your thoughts in the comments below!
Stay Updated
Don’t miss out on the latest advancements in AI. Subscribe to our newsletter for weekly updates on cutting-edge technologies and their impact on the world.
Explore Further
Want to dive deeper into the world of Generative AI? Check out our comprehensive guide on “How Generative AI is Transforming Industries” and discover the endless possibilities.
Summary in a table:
Year | Milestone/Development | Description | Source/Link |
---|---|---|---|
1950 | Turing Test | Alan Turing proposes the Turing Test as a measure of machine intelligence, indirectly influencing the development of systems that could generate human-like outputs. | |
1956 | Dartmouth Workshop | The Dartmouth Workshop is considered the birth of Artificial Intelligence as a field. Early AI research, though not directly focused on generative models, laid some foundational concepts. | |
1966 | ELIZA | Joseph Weizenbaum develops ELIZA, a natural language processing program that simulates a Rogerian psychotherapist. While not strictly generative AI as we know it, it demonstrated early attempts at automated text generation. | |
1980s | Hidden Markov Models (HMMs) | HMMs become popular for sequential data like speech and text, laying some groundwork for later sequence generation models. | |
1990s | Early Neural Networks | Early forms of neural networks, though limited by computational power, begin to explore the potential for learning complex patterns. | (Many resources available – search “history of neural networks”) |
2000s | Rise of Statistical Learning | Statistical learning becomes a dominant paradigm, allowing machines to learn from data without explicit programming, a crucial step for generative AI. | (Many resources available – search “statistical learning in AI”) |
2014 | Generative Adversarial Networks (GANs) | Ian Goodfellow et al. introduce GANs, a breakthrough architecture for generative modeling. | |
2015 | Variational Autoencoders (VAEs) | VAEs emerge as another powerful generative model, offering a different approach to learning latent representations and generating data. | https://arxiv.org/abs/1312.6114 |
2015 | Deep Dream | Google’s Deep Dream algorithm, based on convolutional neural networks, demonstrates the potential for generating artistic and surreal images. | (Many articles and blog posts available – search “Google Deep Dream”) |
2016 | PixelRNN/PixelCNN | Improved models for image generation, paving the way for higher-resolution and more realistic image synthesis. | https://arxiv.org/abs/1606.05328 |
2017 | Transformer Networks | Introduced for machine translation, Transformer networks become crucial for sequence generation tasks, including text generation with models like GPT. | https://arxiv.org/abs/1706.03762 |
2018 | GPT-1 | OpenAI releases GPT-1, a large language model based on the Transformer architecture, demonstrating impressive text generation capabilities. | |
2020 | GPT-3 | OpenAI releases GPT-3, a significantly larger and more powerful language model, showcasing remarkable text generation and comprehension abilities. | https://arxiv.org/abs/2005.14165 |
2021 | DALL-E | OpenAI introduces DALL-E, a model that generates images from text descriptions, demonstrating the power of combining language and vision. | https://openai.com/research/dall-e/ |
2022 | Stable Diffusion | Stable Diffusion is released, an open-source image generation model that becomes widely popular due to its accessibility and performance. | https://stability.ai/ |
2022 | DALL-E 2 | OpenAI releases DALL-E 2, a more advanced version of DALL-E, capable of generating higher-resolution and more diverse images. | https://openai.com/dall-e-2/ |
2023 | Continued Advancements | Rapid progress continues in generative AI, with new models and applications emerging constantly in areas like text, image, music, video, and code generation. | (Many resources available – follow AI research publications and blogs) |
2024/25 | Continued Advancements | Complex prompts, Support for millions of tokens, tool use (ie call external tools, ie search) | Gemini, openAI, deepseek etc. |
Conclusion
The history of Generative AI is a testament to human ingenuity and the relentless pursuit of innovation. From its conceptual origins to the transformative technologies of today, Generative AI has come a long way. As we look to the future, one thing is clear: the journey is far from over. The next chapter in the history of Generative AI is being written right now, and it promises to be even more exciting than the last.